Faster than ever: understanding the accelerating pace of change

In a span shorter than a lifetime, we've leaped from room-sized computers to devices that fit in our pockets, capable of tasks once deemed impossible. We live in a world that is changing faster and faster. Technology has transformed every industry core to our economy over the last few centuries, and today, artificial intelligence is no longer science fiction; it's reality. Adapting to and incorporating these new AI technologies into your company is the critical challenge of this generation of business leaders. To understand where we’re headed, it’s helpful to look at how we got to where we are today.
Computing: the reason for today’s rapid change
While computers are commonplace today, that wasn’t always the case. Computers were invented to automate complex calculations and data-processing tasks. The first electronic computer can be traced back to World War II where it was used to aid in artillery trajectory calculations. After the war, a computer was commissioned by the U.S. Census Bureau to process a large volume of census data and ultimately was able to predict the results of the 1952 U.S. presidential election. Another early adaptation of the computer was the Computer Numerical Control (CNC) machine, which automated the operation of tools such as drills, lathes, mills, and grinders, allowing allowed manufacturers to produce goods faster, with a level of accuracy and precision unattainable through manual methods. Since then, computers have become smaller and faster — primarily due to the increased computational capacity of their chips, enabling a wider range of applications.
In 1965, co-founder of Intel, Gordon E. Moore made the observation that the number of transistors on a computer chip doubles every year, which is now referred to as Moore’s law. Transistors are semiconductor devices for amplifying, controlling, and generating electric signals that make processors more powerful. As the size of each transistor shrinks (due to advancements in semiconductor manufacturing), it allows for more transistors to be packed onto a chip without necessarily increasing the chip's size. This miniaturization allows for smaller electronic devices. Also, smaller transistors switch on and off faster so they consume less power, which saves energy. The chart below shows the increase in transistors per microprocessor over the last 50 years.
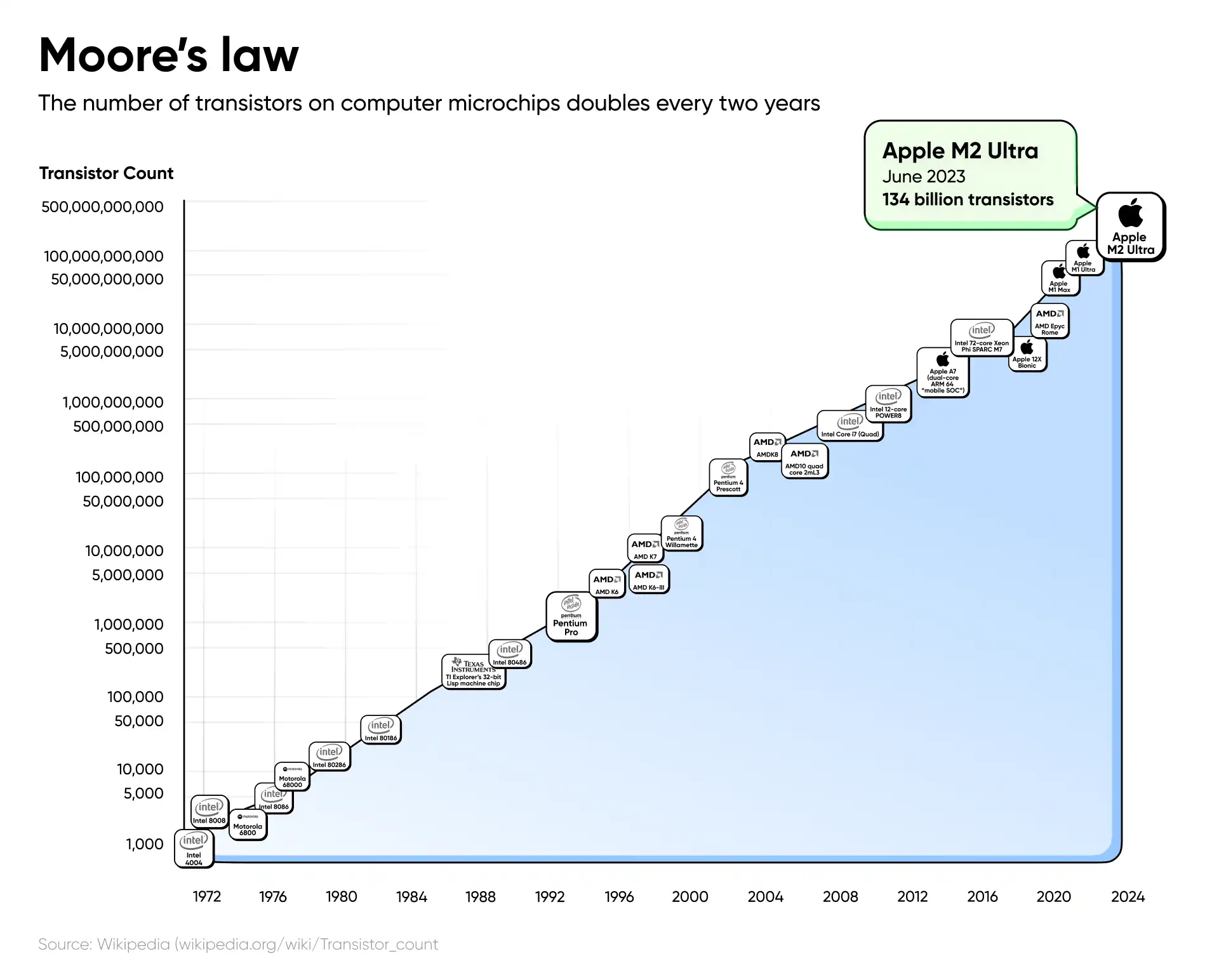
Moore's law has held true for over half a century and has been one of the driving forces behind the rapid advancement of computer technology. The law itself is less of a prediction and more of a coordination metric in the semiconductor industry, setting a predictable two-year innovation cycle. This pushes companies to consistently innovate or risk lagging behind.
While some argue Moore’s law is coming up against the law of physics (transistors now the size of just a few atoms and running up against challenges of power and heat), Intel remains optimistic the growth will stay on course, “aspiring to one trillion transistors in 2030.” Today, Apple’s iPhone 15 Pro has an A17 chip that has 19 billion transistors, each transistor measuring roughly three nanometers. For comparison, a strand of hair is 80,000 nanometers wide.
Computational power increasing at the pace of Moore’s law has enabled large bounds in advanced scientific research, including capabilities in weather forecasting, oil exploration, and protein folding technologies. Continuing to meet Moore’s law could allow for the development of the next set of revolutionary technologies, such as the long-anticipated quantum computer.
Greatest change of our time: the Internet
In the early 1960s, American psychologist and computer scientist J.C.R. Licklider envisioned an “intergalactic computer network” that connected a set of computers for seamless data access. He predicted the use of the computer as a communication device, shifting the computers’ application from just mathematical calculations to something that would “change the nature of communication even more profoundly than did the printing press.” In 1969, UCLA’s Interface Message Processor (IMP) attempted to send the word “LOGIN” to Stanford Research Institute’s IMP and sent the first two letters, “LO,” before the system crashed. Though not entirely successful, this was the world’s first network connection. In 1983, the adoption of a new protocol (the format of data sent over the internet) standardized and simplified communication between different computer systems. Around the same time, IBM introduced its “Personal Computer” and Apple launched “The Macintosh.” As early adopters in the U.S. bought home-computers, they laid the foundation for widespread Internet adoption. To allow for intercontinental internet use, undersea fiber-optic cables were laid across the ocean floor by AT&T Bell Laboratories in 1988. And five years later in 1993, the graphical web browser Mosaic was released, allowing web pages to include both graphics and text — launching an internet adoption boom.

This mass adoption in the U.S., going from roughly 6 million users in 1993 to 180 million in 2003, opened a market for businesses previously unimaginable. Enabled by advancing computer power and the miniaturization of transistors, Apple introduced the iPhone in 2007, putting computers and the internet in our pockets. Since then, we’ve seen mobile communication usher in an era of rapid change, affecting everything, from learning to dating to how we trade on the stock market. Globally, internet usage continues to go up, increasing from just 6% in 2000 to nearly 60% today. Recent advancements in satellite internet, most notably Starlink, are bringing high-speed, low-latency broadband internet service to underserved areas around the world.
While the internet continues to propel the way we live today, the fundamental technology that made the internet possible was the increase in computing capacity over the last 70 years. That computing power — combined with its applications in the internet and software — creates an amount of data so large it’s incredibly difficult to even quantify. In 2010, we produced two zettabytes of data globally. By 2020, that number soared to 64.2 zettabytes. To put that in perspective, one zettabyte is equivalent to roughly 20 trillion 500-page books. Two zettabytes would equate to stacking those books from Earth to the Moon about 2,666 times. And 64.2 zettabytes equate to stacking books from Earth to the Moon over 85,600 times. While not all of this data is useful, there’s an incredible amount of information and patterns waiting to be found, pertaining to some of our largest problems from climate change to public health.

We're now faced with a unique challenge and opportunity: the sheer volume of data available is beyond human capacity to even begin to utilize. Yet, if understood, this data could open doors to incredible opportunities, from predicting disease outbreaks and natural disasters to detecting fraud and optimizing crop yields. The key to parsing this data and unlocking the opportunity it presents is artificial intelligence. While not a new concept, artificial intelligence is useful now more than ever because of the massive amount of data being collected today.
The next greatest change of our time? Artificial intelligence
Artificial intelligence (AI) is “a machine’s ability to perform the cognitive functions we usually associate with human minds.” These functions can include problem-solving, understanding natural language, recognizing patterns, and making decisions. Machine learning (ML) is a subset of AI that’s able to independently edit itself. At its heart, ML operates by taking in sample data provided by an engineer or data scientist, processing it through complex algorithms, learning from the patterns it observes (known as “training”), and then making decisions or predictions based on its own learning. During this process, ML models make predictions and then check them for accuracy against the sample data. The discrepancies between the predictions and the sample data are used to adjust the model's parameters, refining its predictive capabilities over time.

Deep learning takes machine learning one step further by attempting to emulate the human brain — specifically the way biological neurons signal to one another. Deep learning models benefit from vast amounts of data, using it to adjust a large number of parameters, thus they rely on powerful hardware for computation. Deep learning drives innovations like those in autonomous vehicles, where it’s used to detect objects, such as STOP signs or pedestrians. Even Amazon’s Alexa relies on deep learning algorithms to respond to your voice and know your preferences. However, understanding why deep learning models make the specific decisions that they do is often a challenge. Although the algorithms are well-understood, the large number of parameters and complex connections make understanding which factors lead to those decisions difficult to summarize in an easily digestible way.
Artificial intelligence already has a big impact on our daily lives, whether we are aware of it or not. And while AI might seem new, AI research has been around since the 1950s. In the early 1900s, science fiction introduced the idea of artificially intelligent robots, from the "heartless" Tin Man in the Wizard of Oz to Metropolis' humanoid Maria. The field of AI was born from this cultural consciousness, beginning with the “Dartmouth Summer Research Project on Artificial Intelligence” in 1954. It proposed that “every aspect of learning or any other feature of intelligence can in principle be so precisely described that a machine can be made to simulate it.” It was at this time that AI’s exponential growth began.

FLOP (Floating Point Operations Per Second) is a measurement of computer performance. Higher ratings indicate faster performance, which is especially important for training AI systems with a lot of information. In just 70 years, we’ve seen the training computation of AI go from 10 FLOPs to 10 billion petaFLOPs— a trillion-trillion fold increase.
As computational power (measured in FLOPs) increases, AI systems process and analyze larger sets of data more quickly. This enhanced processing capability, combined with the development of improved deep learning algorithms (especially advancements in transformer architectures) and high-quality data, lead to more refined and 'smarter' AI models.
Only a decade ago, the idea of a machine understanding human language or identifying images was almost a fantasy. Yet, by 2015, AI was already matching human levels of image recognition. Today, it far surpasses us. Systems like ChatGPT can understand and generate human-like language at unprecedented scales. Similarly, when given a complex image prompt, AI can generate a detailed picture in just three seconds.
There are many applications of this modern AI technology. Without AI, the 64+ zettabytes of data we have today would be impossible to utilize. As an example, medical professionals have struggled to analyze the enormous volume of patient data generated daily. AI systems can analyze electronic health records, medical imaging data, and even genomic data to identify potential correlations and insights allowing for better disease detection, more personalized treatment plans, and new drug discoveries. Humans could not parse this amount of information alone.
AI is going to be a part of our world moving forward. While it offers many benefits, it also raises concerns related to job displacement and the need for effective regulation. As everyone continues to adopt AI, understanding how it works will be the best way to use it responsibly in the future.
Embracing change
The speed at which the world is changing is so rapid that today's innovation is tomorrow's nostalgia. Blockbuster Video, founded in 1985, was arguably one of the most iconic brands in the video rental space. In 2000, Netflix approached Blockbuster with an offer to sell to Blockbuster for $50 million, as they had very few subscribers. The Blockbuster CEO at the time was uninterested due to Netflix’s operating losses and wrote them off as a "very small niche business". Blockbuster filed for bankruptcy in 2010. In contrast, Netflix grew by adopting new tech as soon as it became available. Most notably, it harnessed user data to develop powerful algorithms for its recommendation system, which now accounts for 80% of time customer’s spend watching Netflix. Netflix is now valued at $167 billion.
Those who embrace change will experience new opportunities for growth. As the world continues to change in unforeseen ways, we recommend manufacturing leaders adapt these practices to adapt to an AI-powered future:
- Reward adaptability. Require, rather than simply encourage, continuous learning and upskilling. Build it into the culture by creating a structured way for cross-sharing these new skills and learnings.
- Act on new technology. Stay informed of new innovations and dedicate time to experiment. Test frequently and move on quickly from wrong choices.
- Choose infrastructure for the future. Invest in infrastructure (systems, software, hardware, etc.) that can fully take advantage of ever-increasing computing power.
- Collect rich data. Create a reliable, accurate body of information that you can use to truly understand your business. With rapid developments in AI, collecting a solid body of data to query and gain insights from will be more important than ever.
Those who embrace the opportunities of technology and innovation have and will continue to thrive, while those who resist will be left behind. Our ability to understand and adapt to these transformative changes will define our future.